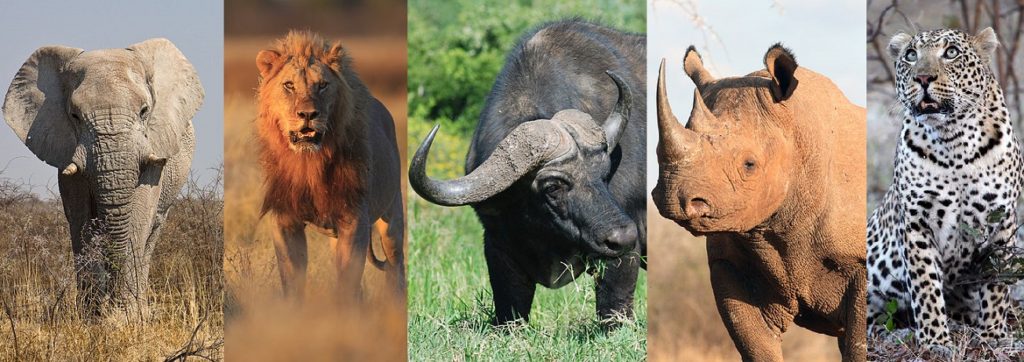
The Big 500 and what it means?
The term ‘Big Five’ originally referred to the difficulty in hunting the lion, leopard, rhino, elephant and African buffalo. These five large African mammal species were known to be dangerous, and it was considered a feat by trophy hunters to bring them home
The term ‘Big Five’ in the early-stage start-up analytics world refers to the difficulty in assessing the Idea/Product, Team, Market Fit, Competition, and Business Model. These five broad components are notoriously difficult to assess due to lack of data, and it is considered a feat by investors to bring home successful exits (over 90% of start-ups fail).
While we can’t claim to be hunters of beautiful animal species (why hunt them?) we can claim to be making in roads on how we hunt the data for the Big Five in early-stage data. Deep research and definition of the factors that lead to both failure and success has allowed the development of our three-score methodology. Algorithms, Deep Dive Experts, and Crowd Wisdom.
Where the Big 500 becomes relevant is that this is a psychological number at which we believe all bias and ego is removed from the system. Use of Distributed Autonomous Organization principals and Network Effects (500 analysts) come into play.
Let me use an example to explain how this works.
Current Problems
- Pain Point 1: Too many Pitches.
Deal Originators such as Crowdfunding platforms, Accelerators, VC companies, Boot Camps, etc. receive hundreds if not thousands of pitches for funding every month and year. For sake of this example lets use the number 100.
- Pain Point 2: Too few analysts
A typical Deal Originator will need a team to analyse the pitches prior to sending them up the chain. Conservatively these initial ‘sifters’ are a small team of 3-5 analysts who are trained to reflect the biases of those up chain. This low number of analysts and too few factors means that ONE analyst can swing a score because they account for 20% of that total score. A Founder doesn’t want their pitch to be the first one of the day (analyst is warming up) nor the last one (analyst is tired). This is how traditional due diligence works. Most Deal Originators collect brief data points and review the Pitches sent to them – Pain Point 3
- Pain Point 3: It’s a Marketing Game
The Founder who does the best sales job and who can appeal to the inherent bias of the Deal Originator stands the best chance of getting to the next stage. The net result is that thousands of ideas are lost to ongoing funding
In our example a typical Deal Originator has only 5 biased analysts reviewing 100 pitches per month, and they are subject to a marketing pitch aka Poor Data. In a 160-hour month (minus breaks) an analyst therefore only spends 1 ½ hour per pitch. From our research, at most they are analysing 20 factors and the rest is gut-feel. That’s a max of 100 answers!
Can you see how GREAT Opportunities are being lost to traditional methods of assessing pitches.
Our book is valuable for anyone who invests, not only in start-ups. Available from Amazon, our with all proceeds from the sale of the book to be used to continue the i3D Platform development and thereafter the payment of analysts.
Don’t miss out on the valuable insights and strategies for building a successful start-up or making smart investments. “Future-Proofing Start-ups” is the go-to resource for entrepreneurs and investors, based on expert knowledge and thousands of hours of research by the i3D Protocol. Act fast to stay ahead of the curve!
Our i3D Protocol Solution
- Solution 1 – Use an algorithm to do the initial ‘Sifting’
No matter the project being looked at among the 100 submitted, the Deal Originator will require some base from which to work. An algorithm can be coded to sift out the pitches that can’t even meet the basic requirements, and it Never, Ever, Gets Tired
- Solution two – Use Crowd Consensus for the initial Idea/Product and Market Fit Assessment
Imagine 500 analysts are very i3D Rapidly just answering 7 Questions on the elevator pitch. That’s 500 X 7 answers. Even if bad actors try to game the system they would need 100 or 20% of the analysts on i3D Rapid to be answering the same way before they had the same affect on the score as defined in the Pain Points above.
- Solution three – use a standardized Deep Dive Methodology for analysts with the highest Reputation
By developing a Reputation Management System it is possible to receive ‘anonymous’ scores on over 50 success factors from a network of ‘invited’ participants. These could be internal and external. It is important that their scores remain anonymous to remove ego and fear of calling out the boss from the process. If the Deal Originator only uses its 5 analysts as defined above, that’s 250 answers!
- Solution four – Code is Law
By using as code and algorithmic processes in the Scoring and Reputation Management Systems it becomes possible to get anonymous and unbiased feedback and remove bad actors from the system. It’s amazing what Machine Learning can do!
- Solution 5 – Rank the opportunities
Once 1-4 are in play, it becomes possible to have all the opportunities ranked and assessed using filters and weighting factors. Imagine the investment decision maker (could be VC or retail) knowing that what they are reviewing is unbiased and ego free from a wide network of analysts. Then Gut-feel can kick in.
There are many more factors that we can use as we build our i3D Protocol, however one thing is obvious. Using the power of A DAO and Network Effects far outweighs the benefit of just keeping Start-up Identification in house. Deal Originators should be keeping this in Mind!
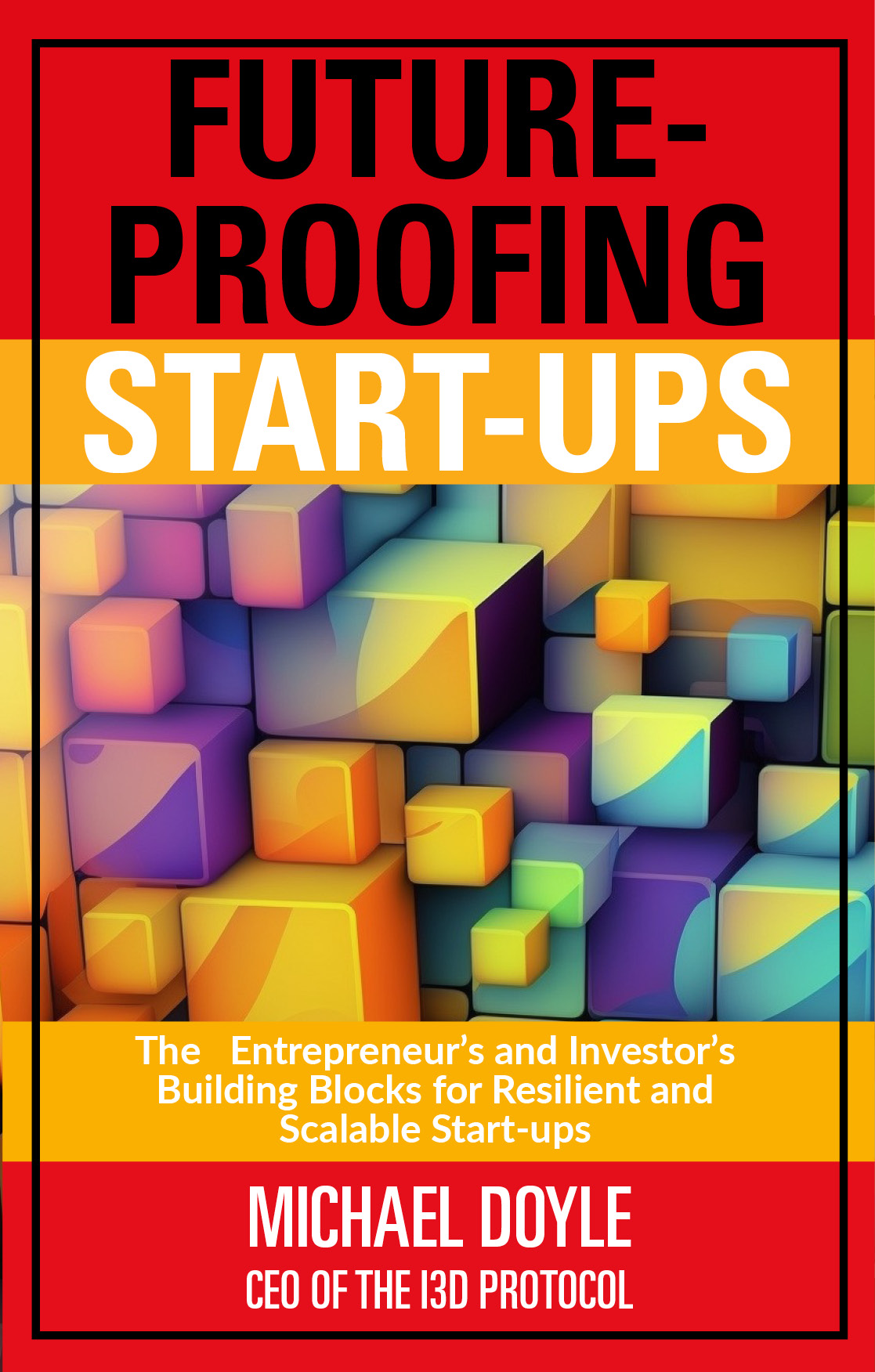
Amazon

https://amzn.to/3DnjI2a